What Nobody Tells You about the Transition to AI Production
- Eitan Netzer
- Dec 12, 2021
- 2 min read
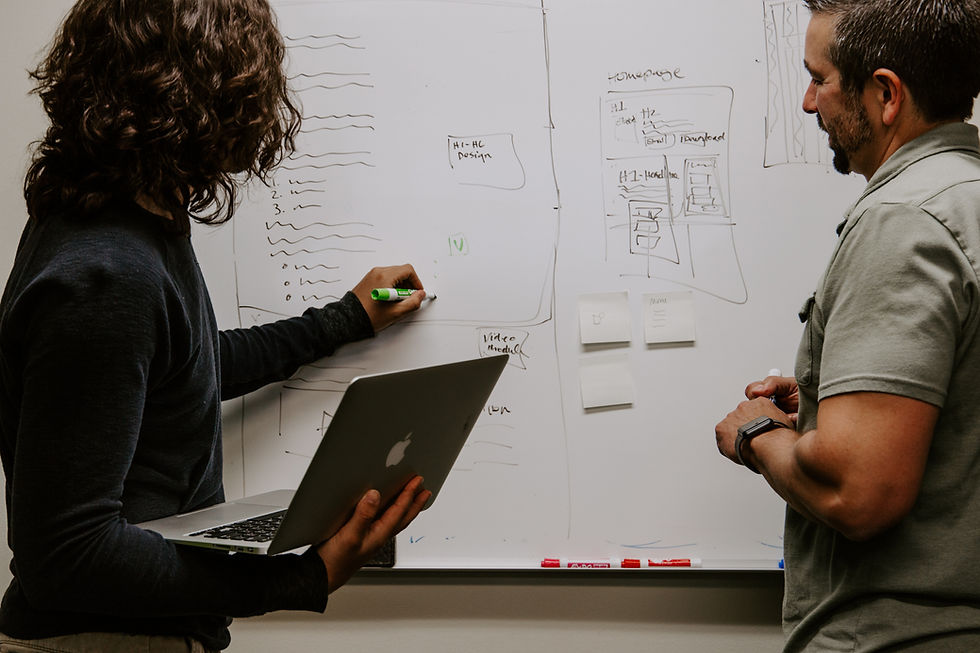
Recently, I participated in a conference dedicated to BI. One of the speakers, a representative of a hardware manufacturing company for AI and ML, stated that almost all companies in Israel are at the AI production stage. According to him, implementing AI is very simple. All you need to do is buy the hardware or server space, download the SDK, run some model training and that's it. You can start production.
But, according to Gartner, 85% of all Big Data projects fail and do not reach the production stage. How can this be if all you need for production is to buy the appropriate server and download an SDK? If it is so easy, why do so many projects fail?
What don't the hardware companies tell you?
The largest AI companies in the market are Google, Nvidia, Amazon, and Microsoft, which employ thousands of AI and ML engineers. However, these companies do not sell AI software solutions. Rather, they sell server space, which their clients sometimes believe is sufficient for production. But this is hardly ever the case.
When transitioning to production, new data is constantly accumulated, and often it is necessary to update the initial AI model. For example, when trying to create a model for consumer behavior, such behavior constantly changes. The model, which was up to date a few months ago, no longer fits the current data and must be updated. AI professionals need to constantly monitor the data to ensure up-to-date models and reliable results. They need to provide ongoing AI maintenance to identify when the model has become outdated and update it on time. Sometimes, this might mean pausing the work of several R&D team members.
This continuous maintenance service requires computing power and working time beyond the initial work and hardware investment. Companies need to be aware of this before entering a new AI project.
For proper maintenance, significant investment in infrastructure is also needed. Additional servers should be bought (or rented from external vendors), and costs might surge without the organization planning or preparing for it. Therefore, it is not enough to consider just the initial investment. Organizations must consider the total cost throughout the entire project life cycle.
Here is what you should know about the transition to production:
The day after going to production, you may occasionally need a data scientist to update the current model (putting his other tasks on hold).
You will need to pay the server provider to increase your account, as you will need greater data capacity and computing power to train the updated model.
You might need to purchase additional hardware.
As the amount of data grows, your data team becomes overloaded, and you may need to hire additional team members - a costly and complicated task in itself.
These are the actual implications of the transition to production. But most organizations are unaware of these implications and do not think about the day after the transition to production. They ask about the project development costs and not about the cost of model maintenance required for adequate service. A successful AI project is not just about the hardware, SDK, or transition to production. In many ways, these are just the beginning.
Comments